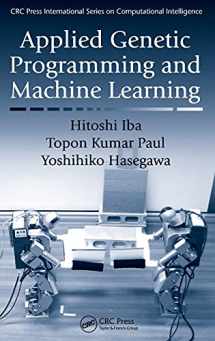
Applied Genetic Programming and Machine Learning (CRC Press International Series on Computational Intelligence)
Book details
Summary
Description
What do financial data prediction, day-trading rule development, and bio-marker selection have in common? They are just a few of the tasks that could potentially be resolved with genetic programming and machine learning techniques. Written by leaders in this field, Applied Genetic Programming and Machine Learning delineates the extension of Genetic Programming (GP) for practical applications.
Reflecting rapidly developing concepts and emerging paradigms, this book outlines how to use machine learning techniques, make learning operators that efficiently sample a search space, navigate the search process through the design of objective fitness functions, and examine the search performance of the evolutionary system. It provides a methodology for integrating GP and machine learning techniques, establishing a robust evolutionary framework for addressing tasks from areas such as chaotic time-series prediction, system identification, financial forecasting, classification, and data mining.
The book provides a starting point for the research of extended GP frameworks with the integration of several machine learning schemes. Drawing on empirical studies taken from fields such as system identification, finanical engineering, and bio-informatics, it demonstrates how the proposed methodology can be useful in practical inductive problem solving.
We would LOVE it if you could help us and other readers by reviewing the book