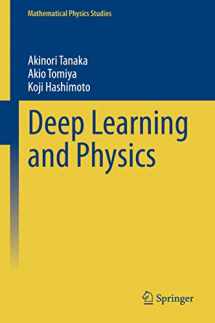
Deep Learning and Physics (Mathematical Physics Studies)
Book details
Summary
Description
What is deep learning for those who study physics? Is it completely different from physics? Or is it similar?
In recent years, machine learning, including deep learning, has begun to be used in various physics studies. Why is that? Is knowing physics useful in machine learning? Conversely, is knowing machine learning useful in physics?
This book is devoted to answers of these questions. Starting with basic ideas of physics, neural networks are derived naturally. And you can learn the concepts of deep learning through the words of physics.
In fact, the foundation of machine learning can be attributed to physical concepts. Hamiltonians that determine physical systems characterize various machine learning structures. Statistical physics given by Hamiltonians defines machine learning by neural networks. Furthermore, solving inverse problems in physics through machine learning and generalization essentially provides progress and even revolutions in physics. For these reasons, in recent years interdisciplinary research in machine learning and physics has been expanding dramatically.
This book is written for anyone who wants to learn, understand, and apply the relationship between deep learning/machine learning and physics. All that is needed to read this book are the basic concepts in physics: energy and Hamiltonians. The concepts of statistical mechanics and the bracket notation of quantum mechanics, which are explained in columns, are used to explain deep learning frameworks.
We encourage you to explore this new active field of machine learning and physics, with this book as a map of the continent to be explored.
We would LOVE it if you could help us and other readers by reviewing the book