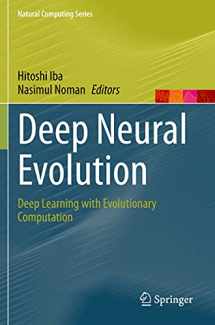
Deep Neural Evolution: Deep Learning with Evolutionary Computation (Natural Computing Series)
Book details
Summary
Description
This book delivers the state of the art in deep learning (DL) methods hybridized with evolutionary computation (EC). Over the last decade, DL has dramatically reformed many domains: computer vision, speech recognition, healthcare, and automatic game playing, to mention only a few. All DL models, using different architectures and algorithms, utilize multiple processing layers for extracting a hierarchy of abstractions of data. Their remarkable successes notwithstanding, these powerful models are facing many challenges, and this book presents the collaborative efforts by researchers in EC to solve some of the problems in DL. EC comprises optimization techniques that are useful when problems are complex or poorly understood, or insufficient information about the problem domain is available. This family of algorithms has proven effective in solving problems with challenging characteristics such as non-convexity, non-linearity, noise, and irregularity, which dampen the performance of most classic optimization schemes. Furthermore, EC has been extensively and successfully applied in artificial neural network (ANN) research ―from parameter estimation to structure optimization. Consequently, EC researchers are enthusiastic about applying their arsenal for the design and optimization of deep neural networks (DNN).
This book brings together the recent progress in DL research where the focus is particularly on three sub-domains that integrate EC with DL: (1) EC for hyper-parameter optimization in DNN; (2) EC for DNN architecture design; and (3) Deep neuroevolution. The book also presents interesting applications of DL with EC in real-world problems, e.g., malware classification and object detection. Additionally, it covers recent applications of EC in DL, e.g. generative adversarial networks (GAN) training and adversarial attacks. The book aims to prompt and facilitate the research in DL with EC both in theory and in practice.
From the Back Cover
This book delivers the state of the art in deep learning (DL) methods hybridized with evolutionary computation (EC). Over the last decade, DL has dramatically reformed many domains: computer vision, speech recognition, healthcare, and automatic game playing, to mention only a few. All DL models, using different architectures and algorithms, utilize multiple processing layers for extracting a hierarchy of abstractions of data. Their remarkable successes notwithstanding, these powerful models are facing many challenges, and this book presents the collaborative efforts by researchers in EC to solve some of the problems in DL. EC comprises optimization techniques that are useful when problems are complex or poorly understood, or insufficient information about the problem domain is available. This family of algorithms has proven effective in solving problems with challenging characteristics such as non-convexity, non-linearity, noise, and irregularity, which dampen the performance of most classic optimization schemes. Furthermore, EC has been extensively and successfully applied in artificial neural network (ANN) research ―from parameter estimation to structure optimization. Consequently, EC researchers are enthusiastic about applying their arsenal for the design and optimization of deep neural networks (DNN).
This book brings together the recent progress in DL research where the focus is particularly on three sub-domains that integrate EC with DL: (1) EC for hyper-parameter optimization in DNN; (2) EC for DNN architecture design; and (3) Deep neuroevolution. The book also presents interesting applications of DL with EC in real-world problems, e.g., malware classification and object detection. Additionally, it covers recent applications of EC in DL, e.g. generative adversarial networks (GAN) training and adversarial attacks. The book aims to prompt and facilitate the research in DL with EC both in theory and in practice.
We would LOVE it if you could help us and other readers by reviewing the book