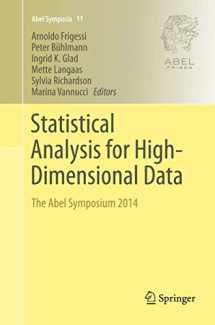
Statistical Analysis for High-Dimensional Data: The Abel Symposium 2014 (Abel Symposia, 11)
ISBN-13:
9783319800738
ISBN-10:
3319800736
Edition:
Softcover reprint of the original 1st ed. 2016
Author:
Sylvia Richardson, Marina Vannucci, Peter Bühlmann, Arnoldo Frigessi, Ingrid Glad, Mette Langaas
Publication date:
2018
Publisher:
Springer
Format:
Paperback
318 pages
FREE US shipping
Book details
ISBN-13:
9783319800738
ISBN-10:
3319800736
Edition:
Softcover reprint of the original 1st ed. 2016
Author:
Sylvia Richardson, Marina Vannucci, Peter Bühlmann, Arnoldo Frigessi, Ingrid Glad, Mette Langaas
Publication date:
2018
Publisher:
Springer
Format:
Paperback
318 pages
Summary
Statistical Analysis for High-Dimensional Data: The Abel Symposium 2014 (Abel Symposia, 11) (ISBN-13: 9783319800738 and ISBN-10: 3319800736), written by authors
Sylvia Richardson, Marina Vannucci, Peter Bühlmann, Arnoldo Frigessi, Ingrid Glad, Mette Langaas, was published by Springer in 2018.
With an overall rating of 4.2 stars, it's a notable title among other
books. You can easily purchase or rent Statistical Analysis for High-Dimensional Data: The Abel Symposium 2014 (Abel Symposia, 11) (Paperback) from BooksRun,
along with many other new and used
books
and textbooks.
And, if you're looking to sell your copy, our current buyback offer is $0.3.
Description
This book features research contributions from The Abel Symposium on Statistical Analysis for High Dimensional Data, held in Nyvågar, Lofoten, Norway, in May 2014. The focus of the symposium was on statistical and machine learning methodologies specifically developed for inference in “big data” situations, with particular reference to genomic applications. The contributors, who are among the most prominent researchers on the theory of statistics for high dimensional inference, present new theories and methods, as well as challenging applications and computational solutions. Specific themes include, among others, variable selection and screening, penalised regression, sparsity, thresholding, low dimensional structures, computational challenges, non-convex situations, learning graphical models, sparse covariance and precision matrices, semi- and non-parametric formulations, multiple testing, classification, factor models, clustering, and preselection. Highlighting cutting-edge research and casting light on future research directions, the contributions will benefit graduate students and researchers in computational biology, statistics and the machine learning community.
We would LOVE it if you could help us and other readers by reviewing the book
Book review
Congratulations! We have received your book review.
{user}
{createdAt}
by {truncated_author}