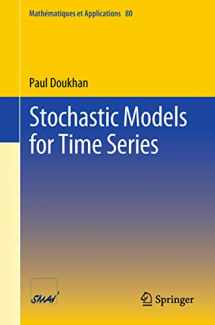
Stochastic Models for Time Series (Mathématiques et Applications, 80)
ISBN-13:
9783319769370
ISBN-10:
3319769375
Edition:
1st ed. 2018
Author:
Paul Doukhan
Publication date:
2018
Publisher:
Springer
Format:
Paperback
328 pages
Category:
Econometrics & Statistics
,
Economics
FREE US shipping
Book details
ISBN-13:
9783319769370
ISBN-10:
3319769375
Edition:
1st ed. 2018
Author:
Paul Doukhan
Publication date:
2018
Publisher:
Springer
Format:
Paperback
328 pages
Category:
Econometrics & Statistics
,
Economics
Summary
Stochastic Models for Time Series (Mathématiques et Applications, 80) (ISBN-13: 9783319769370 and ISBN-10: 3319769375), written by authors
Paul Doukhan, was published by Springer in 2018.
With an overall rating of 3.5 stars, it's a notable title among other
Econometrics & Statistics
(Economics) books. You can easily purchase or rent Stochastic Models for Time Series (Mathématiques et Applications, 80) (Paperback) from BooksRun,
along with many other new and used
Econometrics & Statistics
books
and textbooks.
And, if you're looking to sell your copy, our current buyback offer is $0.3.
Description
This book presents essential tools for modelling non-linear time series. The first part of the book describes the main standard tools of probability and statistics that directly apply to the time series context to obtain a wide range of modelling possibilities. Functional estimation and bootstrap are discussed, and stationarity is reviewed. The second part describes a number of tools from Gaussian chaos and proposes a tour of linear time series models. It goes on to address nonlinearity from polynomial or chaotic models for which explicit expansions are available, then turns to Markov and non-Markov linear models and discusses Bernoulli shifts time series models. Finally, the volume focuses on the limit theory, starting with the ergodic theorem, which is seen as the first step for statistics of time series. It defines the distributional range to obtain generic tools for limit theory under long or short-range dependences (LRD/SRD) and explains examples of LRD behaviours. More general techniques (central limit theorems) are described under SRD; mixing and weak dependence are also reviewed. In closing, it describes moment techniques together with their relations to cumulant sums as well as an application to kernel type estimation.The appendix reviews basic probability theory facts and discusses useful laws stemming from the Gaussian laws as well as the basic principles of probability, and is completed by R-scripts used for the figures. Richly illustrated with examples and simulations, the book is recommended for advanced master courses for mathematicians just entering the field of time series, and statisticians who want more mathematical insights into the background of non-linear time series.
We would LOVE it if you could help us and other readers by reviewing the book
Book review
Congratulations! We have received your book review.
{user}
{createdAt}
by {truncated_author}