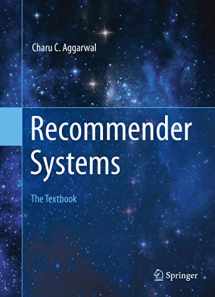
Recommender Systems: The Textbook
Book details
Summary
Description
This book comprehensively covers the topic of recommender systems, which provide personalized recommendations of products or services to users based on their previous searches or purchases. Recommender system methods have been adapted to diverse applications including query log mining, social networking, news recommendations, and computational advertising. This book synthesizes both fundamental and advanced topics of a research area that has now reached maturity. The chapters of this book are organized into three categories:
Algorithms and evaluation: These chapters discuss the fundamental algorithms in recommender systems, including collaborative filtering methods, content-based methods, knowledge-based methods, ensemble-based methods, and evaluation.
Recommendations in specific domains and contexts: the context of a recommendation can be viewed as important side information that affects the recommendation goals. Different types of context such as temporal data, spatial data, social data, tagging data, and trustworthiness are explored.
Advanced topics and applications: Various robustness aspects of recommender systems, such as shilling systems, attack models, and their defenses are discussed.
In addition, recent topics, such as learning to rank, multi-armed bandits, group systems, multi-criteria systems, and active learning systems, are introduced together with applications.
Although this book primarily serves as a textbook, it will also appeal to industrial practitioners and researchers due to its focus on applications and references. Numerous examples and exercises have been provided, and a solution manual is available for instructors.We would LOVE it if you could help us and other readers by reviewing the book