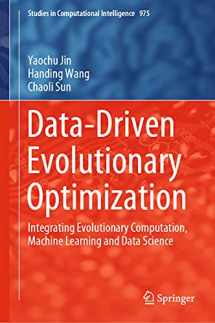
Data-Driven Evolutionary Optimization: Integrating Evolutionary Computation, Machine Learning and Data Science (Studies in Computational Intelligence, 975)
Book details
Summary
Description
Intended for researchers and practitioners alike, this book covers carefully selected yet broad topics in optimization, machine learning, and metaheuristics. Written by world-leading academic researchers who are extremely experienced in industrial applications, this self-contained book is the first of its kind that provides comprehensive background knowledge, particularly practical guidelines, and state-of-the-art techniques. New algorithms are carefully explained, further elaborated with pseudocode or flowcharts, and full working source code is made freely available.
This is followed by a presentation of a variety of data-driven single- and multi-objective optimization algorithms that seamlessly integrate modern machine learning such as deep learning and transfer learning with evolutionary and swarm optimization algorithms. Applications of data-driven optimization ranging from aerodynamic design, optimization of industrial processes, to deep neural architecture search are included.
We would LOVE it if you could help us and other readers by reviewing the book