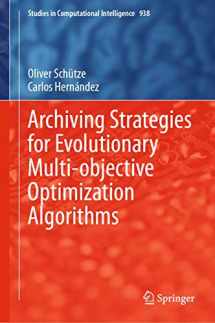
Archiving Strategies for Evolutionary Multi-objective Optimization Algorithms (Studies in Computational Intelligence, 938)
Book details
Summary
Description
This book presents an overview of archiving strategies developed over the last years by the authors that deal with suitable approximations of the sets of optimal and nearly optimal solutions of multi-objective optimization problems by means of stochastic search algorithms. All presented archivers are analyzed with respect to the approximation qualities of the limit archives that they generate and the upper bounds of the archive sizes. The convergence analysis will be done using a very broad framework that involves all existing stochastic search algorithms and that will only use minimal assumptions on the process to generate new candidate solutions. All of the presented archivers can effortlessly be coupled with any set-based multi-objective search algorithm such as multi-objective evolutionary algorithms, and the resulting hybrid method takes over the convergence properties of the chosen archiver. This book hence targets at all algorithm designers and practitioners in the field of multi-objective optimization.
We would LOVE it if you could help us and other readers by reviewing the book