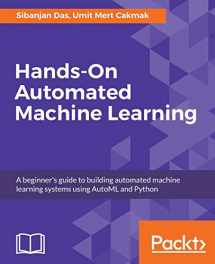
Automated Machine Learning: A beginner's guide to building automated machine learning systems using AutoML and Python
Book details
Summary
Description
Automate data and model pipelines for faster machine learning applications
Key Features- Build automated modules for different machine learning components
- Understand each component of a machine learning pipeline in depth
- Learn to use different open source AutoML and feature engineering platforms
AutoML is designed to automate parts of Machine Learning. Readily available AutoML tools are making data science practitioners' work easy and are received well in the advanced analytics community. Automated Machine Learning covers the necessary foundation needed to create automated machine learning modules and helps you get up to speed with them in the most practical way possible.
In this book, you'll learn how to automate different tasks in the machine learning pipeline such as data preprocessing, feature selection, model training, model optimization, and much more. In addition to this, it demonstrates how you can use the available automation libraries, such as auto-sklearn and MLBox, and create and extend your own custom AutoML components for Machine Learning.
By the end of this book, you will have a clearer understanding of the different aspects of automated Machine Learning, and you'll be able to incorporate automation tasks using practical datasets. You can leverage your learning from this book to implement Machine Learning in your projects and get a step closer to winning various machine learning competitions.
What you will learn- Understand the fundamentals of Automated Machine Learning systems
- Explore auto-sklearn and MLBox for AutoML tasks
- Automate your preprocessing methods along with feature transformation
- Enhance feature selection and generation using the Python stack
- Assemble individual components of ML into a complete AutoML framework
- Demystify hyperparameter tuning to optimize your ML models
- Dive into Machine Learning concepts such as neural networks and autoencoders
- Understand the information costs and trade-offs associated with AutoML
If you're a budding data scientist, data analyst, or Machine Learning enthusiast and are new to the concept of automated machine learning, this book is ideal for you. You'll also find this book useful if you're an ML engineer or data professional interested in developing quick machine learning pipelines for your projects. Prior exposure to Python programming will help you get the best out of this book.
Table of Contents- Introduction to AutoML
- Introduction to Machine Learning Using Python
- Data Preprocessing
- Automated Algorithm Selection
- Hyperparameter Optimization
- Creating AutoML pipelines
- Dive into Deep Learning
- Critical Aspects of ML and Data Science Projects
We would LOVE it if you could help us and other readers by reviewing the book