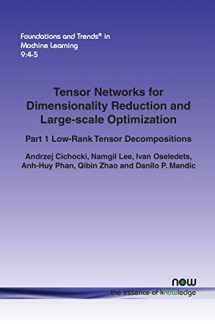
Tensor Networks for Dimensionality Reduction and Large-scale Optimization: Part 1 Low-Rank Tensor Decompositions (Foundations and Trends(r) in Machine Learning)
ISBN-13:
9781680832228
ISBN-10:
1680832220
Author:
Andrzej Cichocki, Ivan Oseledets, Namgil Lee
Publication date:
2016
Publisher:
Now Publishers
Format:
Paperback
196 pages
FREE US shipping
Book details
ISBN-13:
9781680832228
ISBN-10:
1680832220
Author:
Andrzej Cichocki, Ivan Oseledets, Namgil Lee
Publication date:
2016
Publisher:
Now Publishers
Format:
Paperback
196 pages
Summary
Tensor Networks for Dimensionality Reduction and Large-scale Optimization: Part 1 Low-Rank Tensor Decompositions (Foundations and Trends(r) in Machine Learning) (ISBN-13: 9781680832228 and ISBN-10: 1680832220), written by authors
Andrzej Cichocki, Ivan Oseledets, Namgil Lee, was published by Now Publishers in 2016.
With an overall rating of 4.5 stars, it's a notable title among other
books. You can easily purchase or rent Tensor Networks for Dimensionality Reduction and Large-scale Optimization: Part 1 Low-Rank Tensor Decompositions (Foundations and Trends(r) in Machine Learning) (Paperback) from BooksRun,
along with many other new and used
books
and textbooks.
And, if you're looking to sell your copy, our current buyback offer is $0.3.
Description
Modern applications in engineering and data science are increasingly based on multidimensional data of exceedingly high volume, variety, and structural richness. However, standard machine learning and data mining algorithms typically scale exponentially with data volume and complexity of cross-modal couplings - the so called curse of dimensionality - which is prohibitive to the analysis of such large-scale, multi-modal and multi-relational datasets. Given that such data are often conveniently represented as multiway arrays or tensors, it is therefore timely and valuable for the multidisciplinary machine learning and data analytic communities to review tensor decompositions and tensor networks as emerging tools for dimensionality reduction and large scale optimization. This monograph provides a systematic and example-rich guide to the basic properties and applications of tensor network methodologies, and demonstrates their promise as a tool for the analysis of extreme-scale multidimensional data. It demonstrates the ability of tensor networks to provide linearly or even super-linearly, scalable solutions. The low-rank tensor network framework of analysis presented in this monograph is intended to both help demystify tensor decompositions for educational purposes and further empower practitioners with enhanced intuition and freedom in algorithmic design for the manifold applications. In addition, the material may be useful in lecture courses on large-scale machine learning and big data analytics, or indeed, as interesting reading for the intellectually curious and generally knowledgeable reader.
We would LOVE it if you could help us and other readers by reviewing the book
Book review
Congratulations! We have received your book review.
{user}
{createdAt}
by {truncated_author}