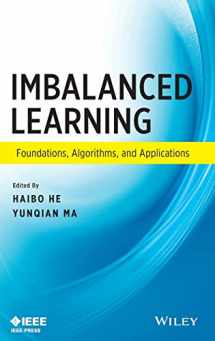
Imbalanced Learning
Book details
Summary
Description
The first book of its kind to review the current status and future direction of the exciting new branch of machine learning/data mining called imbalanced learning
Imbalanced learning focuses on how an intelligent system can learn when it is provided with imbalanced data. Solving imbalanced learning problems is critical in numerous data-intensive networked systems, including surveillance, security, Internet, finance, biomedical, defense, and more. Due to the inherent complex characteristics of imbalanced data sets, learning from such data requires new understandings, principles, algorithms, and tools to transform vast amounts of raw data efficiently into information and knowledge representation.
The first comprehensive look at this new branch of machine learning, this book offers a critical review of the problem of imbalanced learning, covering the state of the art in techniques, principles, and real-world applications. Featuring contributions from experts in both academia and industry, Imbalanced Learning: Foundations, Algorithms, and Applications provides chapter coverage on:
- Foundations of Imbalanced Learning
- Imbalanced Datasets: From Sampling to Classifiers
- Ensemble Methods for Class Imbalance Learning
- Class Imbalance Learning Methods for Support Vector Machines
- Class Imbalance and Active Learning
- Nonstationary Stream Data Learning with Imbalanced Class Distribution
- Assessment Metrics for Imbalanced Learning
Imbalanced Learning: Foundations, Algorithms, and Applications will help scientists and engineers learn how to tackle the problem of learning from imbalanced datasets, and gain insight into current developments in the field as well as future research directions.
We would LOVE it if you could help us and other readers by reviewing the book