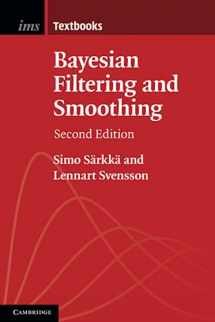
Bayesian Filtering and Smoothing (Institute of Mathematical Statistics Textbooks, Series Number 17)
Book details
Summary
Description
Now in its second edition, this accessible text presents a unified Bayesian treatment of state-of-the-art filtering, smoothing, and parameter estimation algorithms for non-linear state space models. The book focuses on discrete-time state space models and carefully introduces fundamental aspects related to optimal filtering and smoothing. In particular, it covers a range of efficient non-linear Gaussian filtering and smoothing algorithms, as well as Monte Carlo-based algorithms. This updated edition features new chapters on constructing state space models of practical systems, the discretization of continuous-time state space models, Gaussian filtering by enabling approximations, posterior linearization filtering, and the corresponding smoothers. Coverage of key topics is expanded, including extended Kalman filtering and smoothing, and parameter estimation. The book's practical, algorithmic approach assumes only modest mathematical prerequisites, suitable for graduate and advanced undergraduate students. Many examples are included, with Matlab and Python code available online, enabling readers to implement algorithms in their own projects.
We would LOVE it if you could help us and other readers by reviewing the book