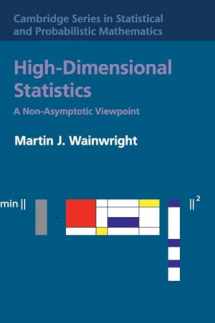
High-Dimensional Statistics: A Non-Asymptotic Viewpoint (Cambridge Series in Statistical and Probabilistic Mathematics, Series Number 48)
Book details
Summary
Description
Recent years have witnessed an explosion in the volume and variety of data collected in all scientific disciplines and industrial settings. Such massive data sets present a number of challenges to researchers in statistics and machine learning. This book provides a self-contained introduction to the area of high-dimensional statistics, aimed at the first-year graduate level. It includes chapters that are focused on core methodology and theory - including tail bounds, concentration inequalities, uniform laws and empirical process, and random matrices - as well as chapters devoted to in-depth exploration of particular model classes - including sparse linear models, matrix models with rank constraints, graphical models, and various types of non-parametric models. With hundreds of worked examples and exercises, this text is intended both for courses and for self-study by graduate students and researchers in statistics, machine learning, and related fields who must understand, apply, and adapt modern statistical methods suited to large-scale data.
We would LOVE it if you could help us and other readers by reviewing the book