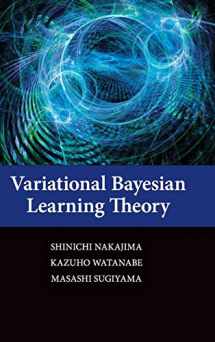
Variational Bayesian Learning Theory
Book details
Summary
Description
Variational Bayesian learning is one of the most popular methods in machine learning. Designed for researchers and graduate students in machine learning, this book summarizes recent developments in the non-asymptotic and asymptotic theory of variational Bayesian learning and suggests how this theory can be applied in practice. The authors begin by developing a basic framework with a focus on conjugacy, which enables the reader to derive tractable algorithms. Next, it summarizes non-asymptotic theory, which, although limited in application to bilinear models, precisely describes the behavior of the variational Bayesian solution and reveals its sparsity inducing mechanism. Finally, the text summarizes asymptotic theory, which reveals phase transition phenomena depending on the prior setting, thus providing suggestions on how to set hyperparameters for particular purposes. Detailed derivations allow readers to follow along without prior knowledge of the mathematical techniques specific to Bayesian learning.
We would LOVE it if you could help us and other readers by reviewing the book