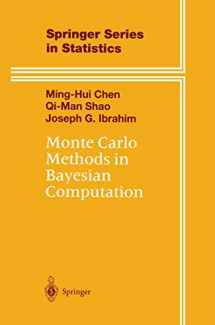
Monte Carlo Methods in Bayesian Computation (Springer Series in Statistics)
Book details
Summary
Description
Dealing with methods for sampling from posterior distributions and how to compute posterior quantities of interest using Markov chain Monte Carlo (MCMC) samples, this book addresses such topics as improving simulation accuracy, marginal posterior density estimation, estimation of normalizing constants, constrained parameter problems, highest posterior density interval calculations, computation of posterior modes, and posterior computations for proportional hazards models and Dirichlet process models. The authors also discuss model comparisons, including both nested and non-nested models, marginal likelihood methods, ratios of normalizing constants, Bayes factors, the Savage-Dickey density ratio, Stochastic Search Variable Selection, Bayesian Model Averaging, the reverse jump algorithm, and model adequacy using predictive and latent residual approaches. The book presents an equal mixture of theory and applications involving real data, and is intended as a graduate textbook or a reference book for a one-semester course at the advanced masters or Ph.D. level. It will also serve as a useful reference for applied or theoretical researchers as well as practitioners.
We would LOVE it if you could help us and other readers by reviewing the book