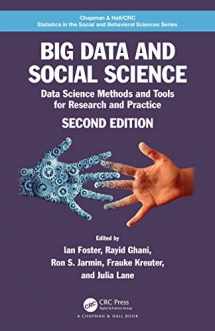
Big Data and Social Science: Data Science Methods and Tools for Research and Practice (Chapman & Hall/CRC Statistics in the Social and Behavioral Sciences)
Book details
Summary
Description
Product Description
Big Data and Social Science: Data Science Methods and Tools for Research and Practice, Second Edition shows how to apply data science to real-world problems, covering all stages of a data-intensive social science or policy project. Prominent leaders in the social sciences, statistics, and computer science as well as the field of data science provide a unique perspective on how to apply modern social science research principles and current analytical and computational tools. The text teaches you how to identify and collect appropriate data, apply data science methods and tools to the data, and recognize and respond to data errors, biases, and limitations.
Features:
Takes an accessible, hands-on approach to handling new types of data in the social sciences
Presents the key data science tools in a non-intimidating way to both social and data scientists while keeping the focus on research questions and purposes
Illustrates social science and data science principles through real-world problems
Links computer science concepts to practical social science research
Promotes good scientific practice
Provides freely available with data, code, and practical programming exercises, through Binder and GitHub
New to the Second Edition:
Increased use of examples from different areas of social sciences
New chapter on dealing with Bias and Fairness in Machine Learning models
Expanded chapters focusing on Machine Learning and Text Analysis
Revamped hands-on Jupyter notebooks to reinforce concepts covered in each chapter
This classroom-tested book fills a major gap in graduate- and professional-level data science and social science education. It can be used to train a new generation of social data scientists to tackle real-world problems and improve the skills and competencies of applied social scientists and public policy practitioners. It empowers you to use the massive and rapidly growing amounts of available data to interpret economic and social activities in a scientific and rigorous manner.
Review
"Like the first edition, the new edition will continue to play an important role for the intended audience and a wider professional community. The much-needed second edition is timely and showcases a wide range of examples and application examples from different areas of the social sciences to demonstrate how the methods are implemented using several real datasets. As expected with this kind of book, the topics of this text are diverse in nature, but interesting none the less. As it is well known, machine learning techniques are subject to inherited bias in model selection and consequently negatively impacts post estimation and prediction. This new edition includes a new chapter on dealing with bias and fairness in machine learning models, a much-needed fair and welcome edition! Further, the authors have done an excellent job in expanding the material on machine learning and text analysis. Like the first edition, the main strength of the book is that it offers a wide variety of applications that are based on real datasets emerging from social science perspectives and useful for both academic and professional communes. As Jupyter has become more popular as the data scientists’ computational notebook of choice, the book has new and improved hands-on Jupyter notebooks to complement each chapter’s material. In conclusion, this new edition has an impressive collection of material on useful and interesting topics on big data. The book will be equally useful to graduate students and researchers interested in gaining perspectives and knowledge on this important topic. The new volume comprises of a wealth of information, a kind of one-stop shop, and can be served as a textbook and research reference book."- S. Ejaz Ahmed, Brock University, Canada
Praise For First Edition
"This book builds a nice bridge connecting social science and big data methodology. Big data such as social media and electronic health records,
We would LOVE it if you could help us and other readers by reviewing the book