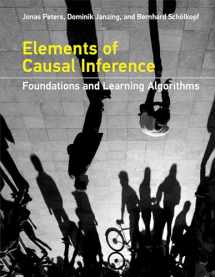
Elements of Causal Inference: Foundations and Learning Algorithms (Adaptive Computation and Machine Learning series)
Book details
Summary
Description
A concise and self-contained introduction to causal inference, increasingly important in data science and machine learning.
The mathematization of causality is a relatively recent development, and has become increasingly important in data science and machine learning. This book offers a self-contained and concise introduction to causal models and how to learn them from data.
After explaining the need for causal models and discussing some of the principles underlying causal inference, the book teaches readers how to use causal models: how to compute intervention distributions, how to infer causal models from observational and interventional data, and how causal ideas could be exploited for classical machine learning problems. All of these topics are discussed first in terms of two variables and then in the more general multivariate case. The bivariate case turns out to be a particularly hard problem for causal learning because there are no conditional independences as used by classical methods for solving multivariate cases. The authors consider analyzing statistical asymmetries between cause and effect to be highly instructive, and they report on their decade of intensive research into this problem.
The book is accessible to readers with a background in machine learning or statistics, and can be used in graduate courses or as a reference for researchers. The text includes code snippets that can be copied and pasted, exercises, and an appendix with a summary of the most important technical concepts.
We would LOVE it if you could help us and other readers by reviewing the book