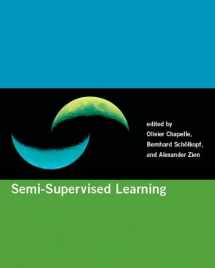
Semi-supervised Learning (Adaptive Computation And Machine Learning)
Book details
Summary
Description
A comprehensive review of an area of machine learning that deals with the use of unlabeled data in classification problems: state-of-the-art algorithms, a taxonomy of the field, applications, benchmark experiments, and directions for future research.
In the field of machine learning, semi-supervised learning (SSL) occupies the middle ground, between supervised learning (in which all training examples are labeled) and unsupervised learning (in which no label data are given). Interest in SSL has increased in recent years, particularly because of application domains in which unlabeled data are plentiful, such as images, text, and bioinformatics. This first comprehensive overview of SSL presents state-of-the-art algorithms, a taxonomy of the field, selected applications, benchmark experiments, and perspectives on ongoing and future research.Semi-Supervised Learning first presents the key assumptions and ideas underlying the field: smoothness, cluster or low-density separation, manifold structure, and transduction. The core of the book is the presentation of SSL methods, organized according to algorithmic strategies. After an examination of generative models, the book describes algorithms that implement the low-density separation assumption, graph-based methods, and algorithms that perform two-step learning. The book then discusses SSL applications and offers guidelines for SSL practitioners by analyzing the results of extensive benchmark experiments. Finally, the book looks at interesting directions for SSL research. The book closes with a discussion of the relationship between semi-supervised learning and transduction.
We would LOVE it if you could help us and other readers by reviewing the book