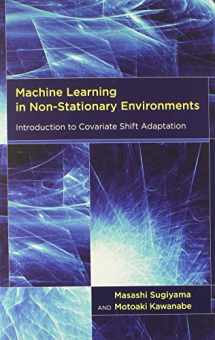
Machine Learning in Non-Stationary Environments: Introduction to Covariate Shift Adaptation (Adaptive Computation and Machine Learning)
Book details
Summary
Description
Theory, algorithms, and applications of machine learning techniques to overcome “covariate shift” non-stationarity.
As the power of computing has grown over the past few decades, the field of machine learning has advanced rapidly in both theory and practice. Machine learning methods are usually based on the assumption that the data generation mechanism does not change over time. Yet real-world applications of machine learning, including image recognition, natural language processing, speech recognition, robot control, and bioinformatics, often violate this common assumption. Dealing with non-stationarity is one of modern machine learning's greatest challenges. This book focuses on a specific non-stationary environment known as covariate shift, in which the distributions of inputs (queries) change but the conditional distribution of outputs (answers) is unchanged, and presents machine learning theory, algorithms, and applications to overcome this variety of non-stationarity.
After reviewing the state-of-the-art research in the field, the authors discuss topics that include learning under covariate shift, model selection, importance estimation, and active learning. They describe such real world applications of covariate shift adaption as brain-computer interface, speaker identification, and age prediction from facial images. With this book, they aim to encourage future research in machine learning, statistics, and engineering that strives to create truly autonomous learning machines able to learn under non-stationarity.
We would LOVE it if you could help us and other readers by reviewing the book