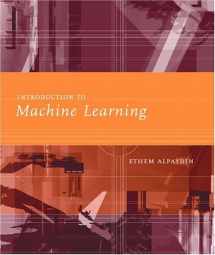
Introduction To Machine Learning (Adaptive Computation and Machine Learning)
Book details
Summary
Description
The goal of machine learning is to program computers to use example data or past experience to solve a given problem. Many successful applications of machine learning exist already, including systems that analyze past sales data to predict customer behavior, recognize faces or spoken speech, optimize robot behavior so that a task can be completed using minimum resources, and extract knowledge from bioinformatics data. Introduction to Machine Learning is a comprehensive textbook on the subject, covering a broad array of topics not usually included in introductory machine learning texts. It discusses many methods based in different fields, including statistics, pattern recognition, neural networks, artificial intelligence, signal processing, control, and data mining, in order to present a unified treatment of machine learning problems and solutions. All learning algorithms are explained so that the student can easily move from the equations in the book to a computer program. The book can be used by advanced undergraduates and graduate students who have completed courses in computer programming, probability, calculus, and linear algebra. It will also be of interest to engineers in the field who are concerned with the application of machine learning methods.
After an introduction that defines machine learning and gives examples of machine learning applications, the book covers supervised learning, Bayesian decision theory, parametric methods, multivariate methods, dimensionality reduction, clustering, nonparametric methods, decision trees, linear discrimination, multilayer perceptrons, local models, hidden Markov models, assessing and comparing classification algorithms, combining multiple learners, and reinforcement learning.
We would LOVE it if you could help us and other readers by reviewing the book